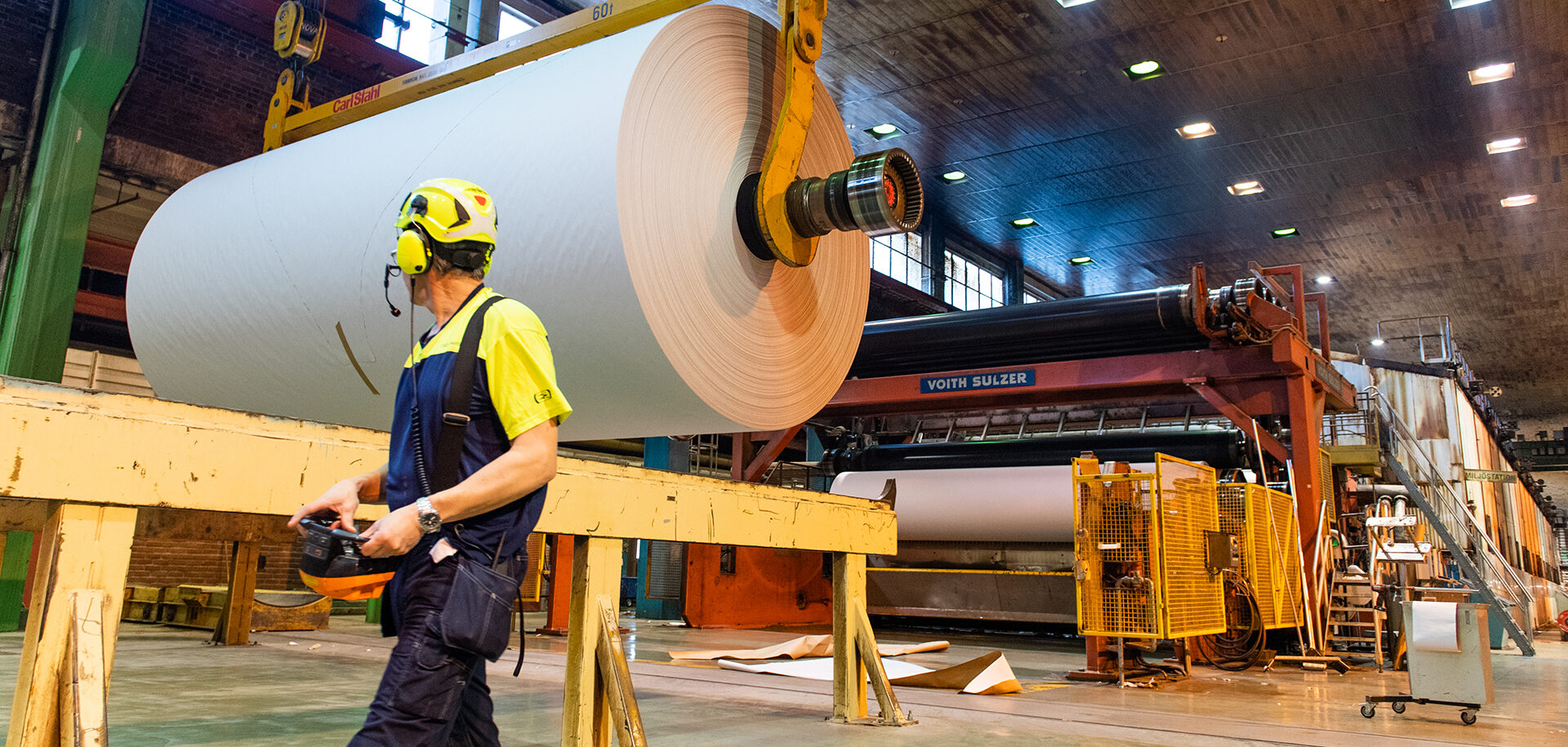
Condition Monitoring with AI at Your Elbow
A research project at Luleå University of Technology in northern Sweden, in collaboration with SKF, is bringing the power of generative artificial intelligence to the world of machine reliability.
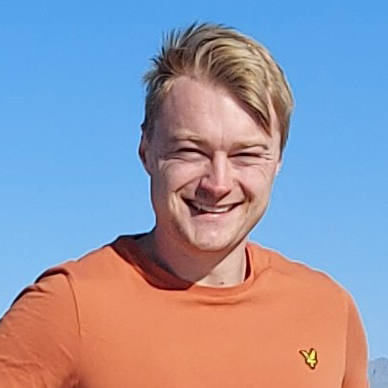
Advanced condition monitoring technology has changed the way industrial companies manage and maintain their assets. By analysing machine parameters such as vibration levels, temperature variations and power consumption, operators can get an early indication of potential problems. This helps them plan and execute timely maintenance interventions to maximize uptime and keep production on track.
The automation of condition monitoring is difficult, however. “The relationship between vibration signals and certain bearing faults is well studied and understood in a laboratory,” says Karl Löwenmark, a PhD student at Luleå University of Technology. “But doing the same in a production environment is much more challenging and requires an application engineer with years of experience.”
Löwenmark’s industrial sponsors include some of the world’s most demanding users of condition monitoring technology. In addition to SKF, there are two large paper mills, each of which operates huge paper-making machines equipped with thousands of sensors. “The machines I work with can be tens of metres long,” he explains. “A pulp and paper machine consists of many different rotating parts, which may run under harsh conditions including high humidity and temperature. As a result, a significant amount of information is hidden in the sensor signals.
The use of AI in the automation of bearing condition monitoring has significant potential,
Cees Taal, senior researcher, SKF Research and Technology Development
“Diagnosing problems in the signals from the machines requires a lot of skill and expertise,” Löwenmark continues, “but the sheer volume of data can be overwhelming.” In the worst case, this means that analysts must spend several hours each day sifting through false alarms and other erroneous data before they can even begin to identify and diagnose potential machine problems.
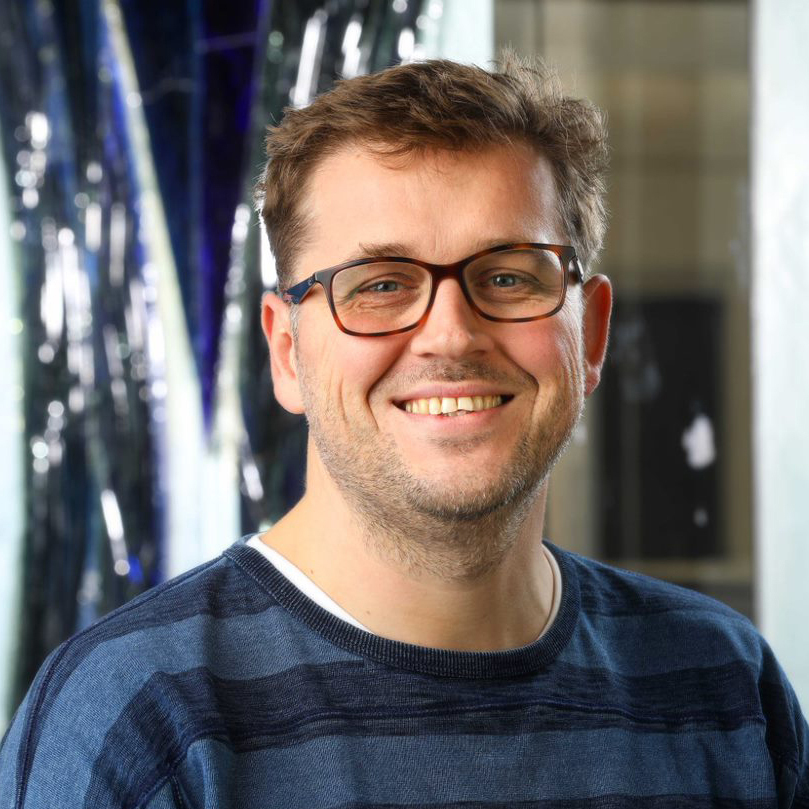
Löwenmark’s PhD project, which began in 2020, aimed to explore how emerging AI technologies could ease the burden on these expert analysts, freeing them to spend more time on the value-added activities that help paper mills run more reliably. This would remove a key barrier to wider adoption of condition monitoring, as the people with the knowledge and experience to operate these systems are often in short supply.
“The use of AI in the automation of bearing condition monitoring has significant potential,” says project co-sponsor Cees Taal, a senior researcher in the diagnostics and prognostics team, SKF Research and Technology Development, Houten, the Netherlands. “However, applying these concepts in this domain is less straightforward, as in the field of vision and language.”
“My project coincided with a period of dramatic advances in AI technologies,” Löwenmark explains. “When I started, large language models like OpenAI’s GPT system were in their early stages of development and not as publicly accessible as they are today. Much of my work has been about keeping up with the progress that has been made in the past four years, then finding ways to transfer this progress to industry-specific challenges.”
From images to signals
Classifying or detecting objects in an image has made a significant leap in the past decade. However, applying these methods to condition monitoring data to diagnose or prognose a bearing fault is far from trivial. The first challenge is to tell the AI model what to look for. “These systems are trained on huge amounts of data,” Löwenmark explains, “The AI companies build huge training data sets using carefully labelled and categorized examples from the internet. Condition monitoring data, on the other hand, is plentiful but challenging to utilize, as it is unstructured, heterogenous and, most importantly, unlabelled.”
Löwenmark did have some resources, however. Over the years, condition monitoring experts at SKF and the two paper mills had accumulated hundreds of reports documenting machine failures, corrective actions and the data used to diagnose them. The plan, he says, was to use these reports to train a general-purpose AI model to handle condition monitoring data.
It wasn’t easy. “Condition monitoring reports are very different from the typical text and images used to train AI models,” Löwenmark says. “The data can be presented in many different ways, and the language used by analysts is very technical and includes industry- and company-specific jargon that is rarely used elsewhere.”
On the “image” side, the system is designed to handle the complexity of condition monitoring signals. “If you train an AI to recognize a picture of a dog, it’s easy because you know there’s a dog in the training image,” he says. “In monitoring, signals can be transient and evolve over time, so you need to look at historical data in addition to the current signal and maybe look at different representations of that data as well.”
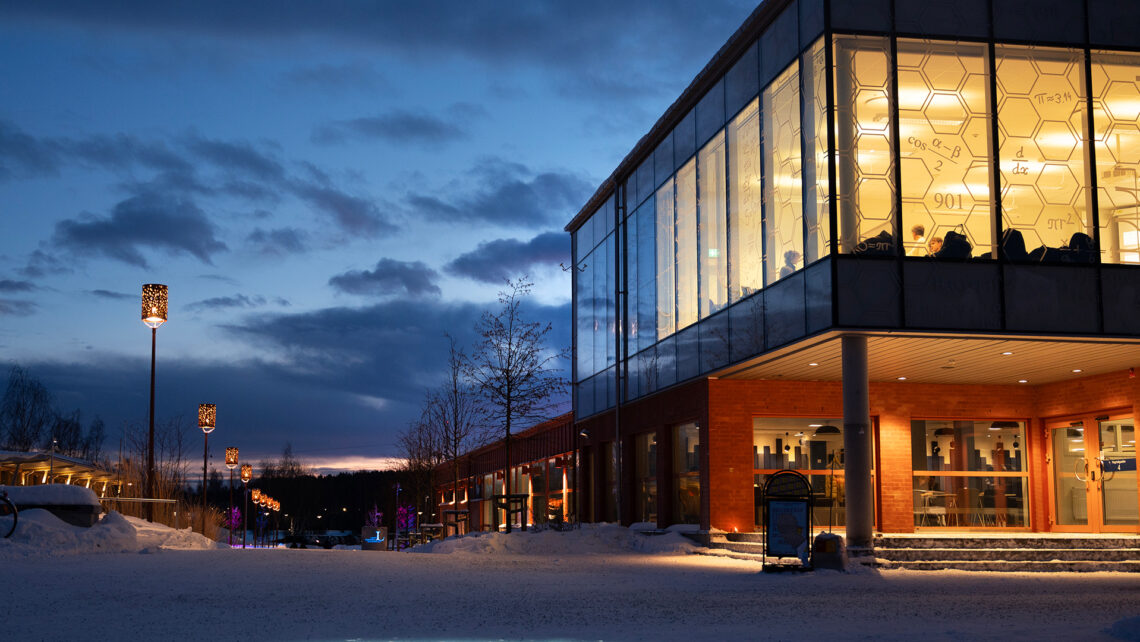
Making sense of technical language
Solving these problems required ingenuity. Löwenmark used an array of techniques to convert the engineers’ data and reports into a format that today’s AI systems could interpret. He included explanations of the data and definitions of key terms in the prompts used to run the AI model and provided the system with links to explanatory documents.
Löwenmark used these elements to fine-tune “dual supervision” AI models. This type of system is designed to process text and image data simultaneously. It is the technology used to automatically generate descriptions of photos or video clips or to create synthetic images from user-provided prompts.
The new models quickly proved their ability to handle real-world condition monitoring tasks. An early success came when Löwenmark showed that an AI model could reliably recognize signals generated by faults in sensors or sensor cables. These events are relatively common in large condition monitoring installations. They are easy to fix but finding them in the data typically requires time-consuming review by a human analyst.
“If an AI can identify these faults, the analyst only needs to review and confirm the diagnosis,” he says. “This would be a useful time saver.”
The AI assistant is evolving
AI can also help diagnose more complex problems. Newer versions of Löwenmark’s system are designed to help analysts do this work faster and more accurately. “In one example, an analyst can look at a signal and start typing a description of the problem,” Löwenmark says. “As they do that, the system can automatically pull up examples of similar events that have happened in the past, showing what the data looked like and what the problem was. That can help the analyst with their own diagnosis or point them to a colleague who has more experience diagnosing similar problems.”
The next iteration of the system, currently in development, aims to extend the AI model into an interactive virtual assistant for condition monitoring analysts. It will feature a “chat” interface that can respond to text queries from the user, pull up relevant data, run analytical tools and suggest the next steps needed to diagnose a problem.
Does this mean that AI condition monitoring is ready for prime time? Löwenmark is optimistic, but cautious. “I would say we have a robust framework, but every condition monitoring installation is different, so users would need to train the models with their own data and refine them to match their policies and processes. AI systems can use reinforcement learning, so the model gradually improves the relevance and accuracy of its results based on feedback from the user.”
SKF is currently investigating the transferability of his approach. Discussions are underway on a new project to apply the AI approach to other applications.
Karl Löwenmark’s work is supported by the Strategic innovation programme Process industrial IT and Automation (PiIA), a joint investment of Vinnova, Formas and the Swedish Energy Agency. In addition to Luleå University of Technology and SKF, the project also involves the research institute RISE, and paper mills SCA Munksund and Smurfit Kappa Piteå.