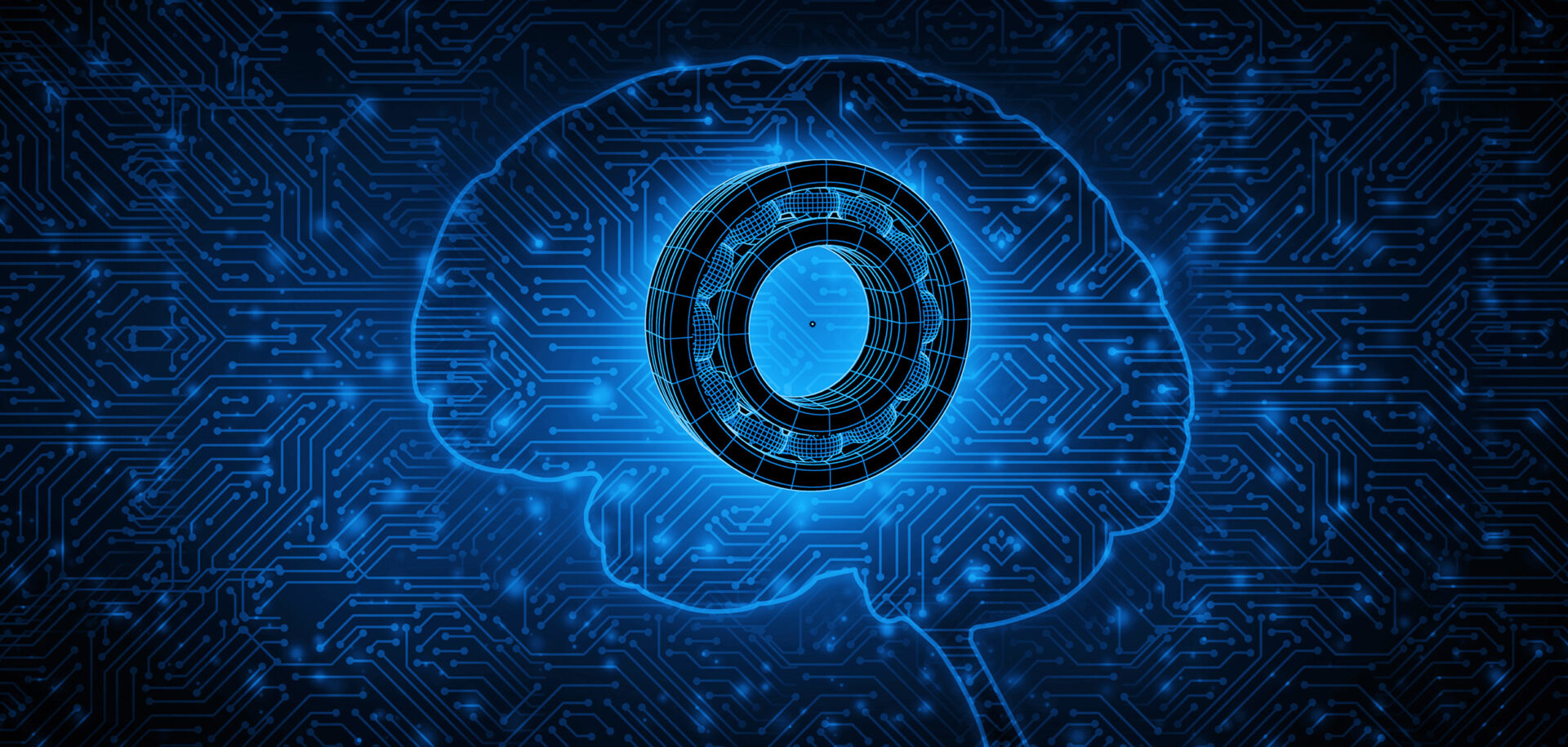
The future of bearing failure analysis is here
Computer vision is an effective complement to the human eye. SKF has developed an AI tool to evaluate bearing damage automatically, helping customers diagnose problems and extend the life of critical components.
Industrial users increasingly rely on advanced digital technologies to monitor the health of their equipment. Modern condition monitoring systems apply advanced analytical techniques to detect the early signs of wear or damage in bearings and other critical parts. Once an issue has been identified, however, the most powerful diagnostic tool has always been the eyes of an experienced engineer.
By looking at tell-tale marks on contact surfaces, SKF specialists can evaluate the severity of a problem and often identify its most likely cause. That information is hugely valuable for end users. For example, visual inspection may allow them to modify operation or maintenance procedures to prevent problems from reoccurring. It can also determine, in the case of the large bearings used in applications such as paper mills, steel production or wind turbines, whether a damaged bearing is suitable for remanufacturing and reuse.
Accessing a skilled bearing analyst isn’t always easy, however. It can take years of working with bearings every day for SKF specialists to accumulate the necessary knowledge and experience. If the damaged equipment is in a remote location, just getting a bearing engineer on-site can be difficult, a problem compounded in recent months by restrictions on travel and access imposed in response to the Covid-19 pandemic.
In addition, when used bearings are due to be sent back to SKF for remanufacture, owners want to know that the item they are shipping is in suitable condition to be recycled. If it isn’t, it will probably be more cost-effective and environmentally friendly to recycle the bearing locally.
AI system for evaluating bearings
To address these issues, a team at SKF is developing a new approach. They have built an automated computer vision system that can evaluate bearing damage using digital photographs. The system uses artificial intelligence in the form of a neural network image-recognition algorithm that has been trained using thousands of images of damaged bearings from SKF’s archives.
The system can identify bearing surface features even if the image is shot at an imperfect angle or the background is cluttered.
Unlike older machine-vision approaches, the AI vision system has been designed to operate in real-world conditions, where images may be obtained on the factory floor or in the field, and tight control over lighting and composition are impossible. The system can identify bearing surface features even if the image is shot at an imperfect angle or the background is cluttered.
Once it has picked out the area of interest, the AI tool classifies the type and severity of damage on the bearing surface. Bearings can exhibit many different types of failure. For example, ISO 15243:2017, the relevant international standard, lists more than a dozen. The SKF team chose to focus for now on a select number of failure modes that account for around 80 % of failures in service.
Training the algorithm
Training and optimizing the algorithm was a collaborative effort between a team of researchers at SKF Research and Technology Development Center and a group of highly experienced bearing analysis specialists. First, the team showed the system thousands of images, each tagged with the relevant failure mode, to teach it the characteristics of each failure mode. Then, to test the algorithm, they asked it to classify new images and compared its results to the views of the human experts, tweaking the model to improve its accuracy.
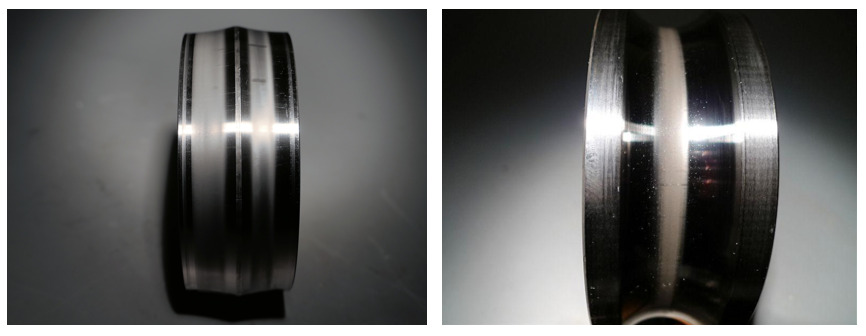
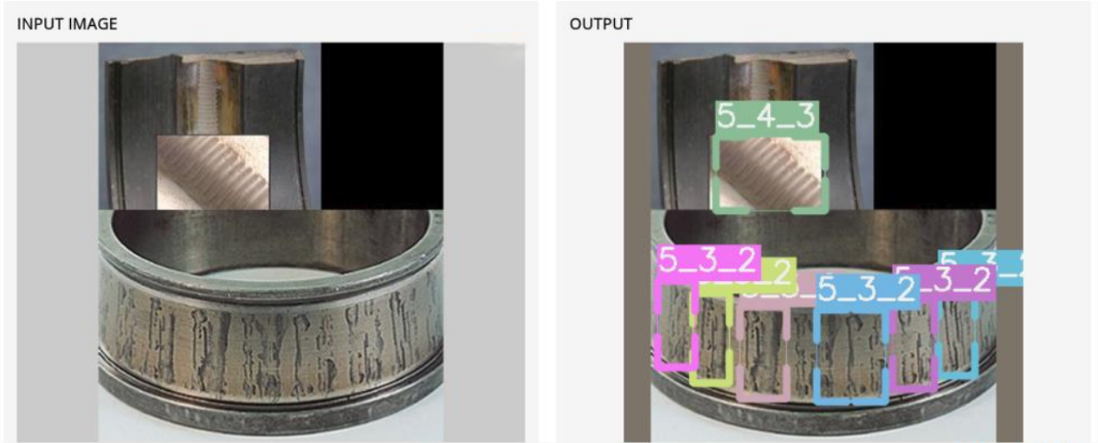
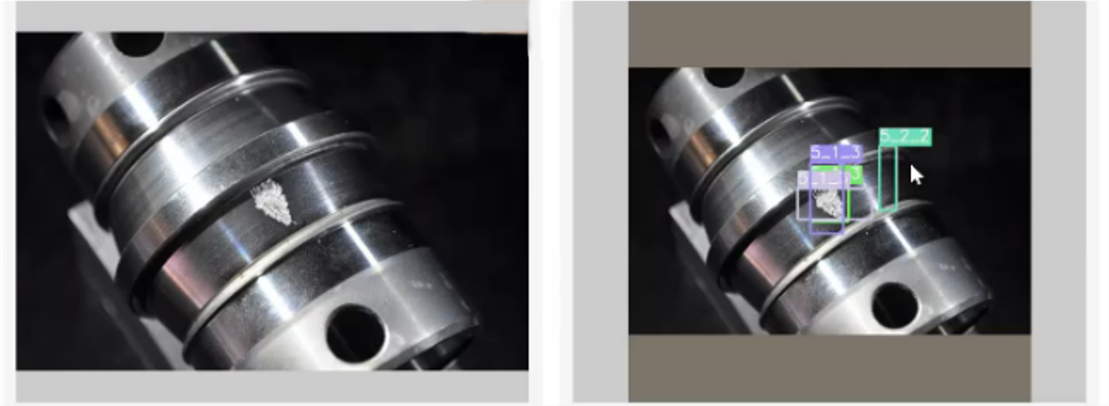
Deployment and continuous learning
With the AI vision system performing well during the testing phase deployment has started across the organization to help customer support teams classify problems and remanufacturing personnel accelerate the evaluation of incoming bearings. Working alongside experienced people, the computer vision algorithm continues to learn, improving its accuracy all the time.
The next phase of the project will be to make the system available to customers. The team plans to deploy it as cloud-based software, allowing maintenance people to take pictures of bearings and upload them to the cloud for analysis. The initial goal is a seamless AI-augmented experience that instantly helps a user decide if a bearing is suitable for remanufacture.
Further down the line, SKF is exploring ways to combine the new approach with other analytical tools in its portfolio. Knowing how a bearing failed does not tell you why it failed, for example, since the same problem can arise from multiple root causes. But by linking AI vision with data collected from condition monitoring and machine control systems, the team envisions to automate and accelerate root-cause problem solving and the resolution of reliability challenges.